Top creatives at Technicolor, Foundry, and Framestore discuss machine learning and how it will impact VFX workflows in the years to come. Read on to learn why machine learning will streamline, rather than replace, creativity.
Could a machine ever replace human creativity? With headlines like “Will AI write the next great American novel” and “Movie written by algorithm turns out to be intense” there’s reason to speculate. However, when it comes to visual effects workflows, AI is less likely to take over the entire creative process and more likely to support more efficient and capable VFX workflows. How? By taking care of the drudgery most people usually don’t want to do!
Below, top technologists at VFX studios and software companies discuss machine learning. Their sentiment is aligned: machine learning is likely to be a time-saver for VFX creatives who build, assemble, and blend effects in post-production and real-time. Indeed, as evidenced by the recent ACM SIGGRAPH and DigiPro talks on the subject, machine learning is already starting to improve efficiencies and workflows for clients, artists, and customers around the globe.

Dan Ring, Head of Research at Foundry
First, what do we mean when we refer to Artificial Intelligence (AI), machine learning (ML), and deep learning (DL)—terms often used interchangeably?
“In a nutshell, AI is the general catch-all term for computers making reasoned decisions about something,” says Dan Ring, Foundry’s Head of Research. “Machine learning is a subset of AI, where you use data to build a model (or train a model) that informs what will happen given a signal. Deep learning is a type of machine learning, where the model for making the decisions (or inference) can be far more complex, with orders of magnitude greater than non-deep models.”
For example, given a signal, a video game AI might choose to turn left or right at a crossroads. “With machine learning, you might teach the character to turn left or right based on whether previous decisions have generated a reward or not,” says Dan. “A single, non-deep neural network—one created perceptron-style from linear machine learning algorithms used for binary classification tasks—might have one or two ‘layers of neurons’. A deep network would have 80+ layers.” This leap from simple machine learning to deep learning has been made possible by recent advancements in general processing on GPUs, he adds.
Foundry started using simple, non-deep machine learning inside its Oscar-winning suite of 2D image-processing plug-ins, Furnace. “Machine learning in Furnace enabled unsupervised learning/clustering for keying and motion modeling,” says Dan. “That helped with complicated tasks and made frame-by-frame tasks, like painting out objects, much easier.”
Dan and his team launched a new deep approach to machine learning a few years ago. “We saw previously impossible tasks, like deblur and semantic masking, suddenly tackled with relative ease,” he says. “We were so impressed that we wanted to get these features into the hands of artists immediately.” Using an open-source machine learning server to navigate engineering barriers, Foundry’s approach allowed more adventurous users to implement deep learning on their data. You can read more about this in Dan’s co-authored ACM Siggraph paper on the subject, Jumping in at the deep end: how to experiment with machine learning in post-production software.
Awareness of machine learning has risen steadily since Dan authored the paper. He says this growing curiosity fed a developing appetite among VFX software users to experiment with machine learning and solve unique workflow problems. “For this reason, Foundry built CopyCat; a tool inside of Nuke that wraps up the common machine learning training workflows and allows users to create and use models on their data,” he explains.
Although CopyCat can streamline and optimize many processes, the tool has put more options at artists’ fingertips; not threatened their livelihoods. “From what we’ve seen so far, I don’t think AI will replace what artists can do anytime soon,” says Dan. “CopyCat has put the power of machine learning into artists’ hands, rather than taking it from them. Ultimately, machine learning has empowered artists to remove drudgery and repetitive tasks for their workflow and focus on being creative. And being creative is exactly where they should place their talents.”
Dan emphasizes that much of the work in VFX machine learning today, across the industry, is focused more on reducing time-consuming, repetitive tasks than replacing the creative process itself. “Machine learning in VFX is used for things like Denoise, better indexing and the retrieval of assets, and things like neural rendering for performance transfer. A great example of that is when Digital Domain created the Vince Lombardi digi-double for the Super Bowl, using its Charlatan machine learning process.
“At Foundry, we also recently completed a project with DNEG around accelerating roto called SmartROTO. What’s important to remember is that while we certainly made advancements, good roto is super hard to do. AI may be able to speed up parts of it, but the experience and knowledge needed to deliver industry-standard roto remain with the artists.”
Dan sees even more potential for machine learning in pipeline and production-focused tools, such as AI tools that can spot errors in renders, predict job timings, estimate bid costs, and more. Foundry is also actively investigating how to use machine learning tools on real-time virtual sets and has already started testing CopyCat for use in virtual production workflows.
Benoit Maujean, Global Head of Research at Technicolor
Technicolor is also invested in using machine learning to save its artists’ time and create better imagery. Currently, Technicolor is focused on data-driven automation for VFX and animation across the pipeline, from color grading and image processing to 3D reconstructions, matchmoving, roto, and animation style transfers.
MPC, MR. X, Mikros, and The Mill all come under the Technicolor umbrella, and Benoit Maujean leads research across each. “We’re currently building a new technology pipeline with computer vision techniques to create a 3D panoramic reconstruction of the shooting set,” he explains. “Right now, we’re doing this with Meshroom, an open-source 3D scanning and reconstruction software based on the AliceVision Photogrammetric Computer Vision framework developed at Mikros Image before it was acquired by Technicolor in 2015. Ultimately, however, all of this will be achieved automatically using machine learning.”
Benoit says machine learning will potentially improve the quality of the data collected. “Getting all of this data is a complex problem, and it’s sometimes difficult to get the details of a texture. However, machine learning will improve the robustness and speed of these processes.”
Although AI, machine learning, and deep learning are generally accepted as valuable additions to the VFX pipeline, this wasn’t always the case. Benoit attributes this to the technology’s lack of visibility—something that is integral to creative work. “In the beginning, machine learning was considered a black box,” he says. “Machine learning either worked, or it didn’t. When it didn’t work, it wasn’t easy to tweak the parameters to get the result you wanted with any precision. However, when you mix machine learning with computer vision techniques as we are today, the process is no longer a black box; it’s more like a kind of toolbox that’s integrated into your global pipeline.”
The longer game, says Benoit, is completely automating ingestion of machine learning data, including capture devices like LiDAR, from live-action cameras on set. “Right now, processes like 3D reconstruction, panoramas, match-moving, and 3D roto animation are done manually. For example, a matchmove takes about half a day to two days; a single roto shot can take up to a week. However, in the future, the great majority of shot ingestion will be automatic, and with new machine learning tools, we will progressively learn to what extent we can increase the number of shots that can be batch processed.”
Technicolor has several ambitious machine learning research projects underway, including transferring live-action capture of actors and facial expressions onto rigs created by animators. “We’re also looking at how machine learning will help our artists be even more creative,” says Benoit. “One way we will be able to do that is through automation, saving artists’ time but also empowering them to start on creative VFX earlier in the process. Machine learning also enables the robust and consistent 3D representation you need to build complex, magnificent VFX.”
Katalina Williams, Head of Software, Advertising at Technicolor
Katalina Williams heads up software at Technicolor’s advertising divisions MPC and The Mill. She’s also the lead developer and product owner of the division’s ftrack Studio integration, with a mandate to streamline project management.
“We’ve provided most of our advertising-specific machine learning to artists via ftrack Studio,” says Katalina. “We’ve implemented several ftrack Actions, for example. One Action automatically generates mattes for a roto based on a plate input. Someone will publish the plate to ftrack when they conform the timeline for the job, and an option generates the mattes.”
Katalina is keen to underline that such processes are about efficiency, not replacing creative work. “We’re not trying to eliminate positions or anything like that,” she continues. “However, the work we’ve done makes it easy to roll first-pass versions to clients quickly, and that’s been huge. The people we’ve introduced this process to have been amazed that they can work so fast. When you look at the situation from that perspective, there’s nothing to be worried about.”
Johannes Saam, Senior Creative Technologist at Framestore
When working at Scanline VFX in 2014, Johannes Saam won a Technical Achievement Academy Award for developing prototype workflows for deep compositing that used depth data to composite images. He has since branched out to apply the knowledge to a broader range of special projects; everything from rides and commercials to virtual reality and virtual production.
“At Framestore, we are creating something very unique in VFX: a lot of annotated data with lots of additional information connected to that data,” he says. “We’re spitting out complex images constantly. Many of us want to figure out ways to capitalize on that data and work more efficiently. Machine learning helps us to do that.”
One area in which Framestore is currently investigating machine learning is rigging. Framestore is known for its CG creatures, so the studio has been exploring how machine learning can provide artists with more close-to-real-time animation rigs—with all muscles and skeletal systems articulated—much earlier in the creative process. “Automating rigging is a classic example of taking something that usually takes a lot of time to build and render, then using the talent and data we already have to speed up the process.”
Johannes, a futurist with his hands in several AI research projects on any given day, says this is just the beginning. “I think machine learning is going to start happening everywhere, whether in rigging or rendering or whatever,” he says. “There are a billion possibilities. You can go to any point in the pipeline and find some time-consuming process that involves a lot of data, and we’ll be able to take it, bake it, and make it think.”
Fredrik Limsäter, CEO at ftrack
“Last year, I spoke of machine learning’s potential to open up new pathways for creative work and bring more adaptive, agile workflows to production pipelines,” says Fredrik. “I still believe this is an integral part of the future of visual effects and, alongside innovations like real-time, will be the next inflection point in how we create and what we create.
“The opportunities are boundless. Using machine learning, we could constantly track and analyze a studio’s output over a year, then use that information to suggest the best possible bid for a particular type of work. For example, what should a bid be for a project that contains a certain amount of animation, a certain amount of compositing, and so on; ftrack Studio could accurately answer that question based on objective, empirical data. Likewise, we could mine data to see how long a specific type of project might take, or we could reveal what delays one kind of project is more likely to suffer as compared with other project types. Then, we could extrapolate from that information to forecast required workloads and timings across a project. Such information would be hugely beneficial to studios working with razor-thin margins.

“We also have Actions at ftrack. Using Actions, we could parcel up specific machine learning processes and then offer them to our users. So if there is a particular process that machine learning would help to expedite, users could activate it at the click of a button and shave minutes or even hours off their daily schedule. We could even use machine learning to suggest automated Actions that might speed up the manual processes of a studio workflow, or run tooltips that suggest more efficient ways of working based on millions of data points.
“The possibilities are endless and so exciting to consider. We’re looking into all of the above and more at ftrack. Ultimately, whatever we do with machine learning, the result should be that producers can set up and track projects with much more accurate insight. With the time they win back, their studios can take on truly ambitious projects and create more stunning work for us to watch on the big screen.”
Try ftrack today
Automate repetitive processes so your team can do what they do best: create.

More from the blog

Enhanced performance in ftrack Studio: Fine-tuning for speed, reliability, and security
Chris McMahon | API, Developer, New features, Product, Productivity, Studio | No Comments
Backlight and the Visual Effects Society forge a partnership for the VES Awards judging process
Kelly Messori | Case Study | No Comments
Presenting the new sidebar: Enhancing project navigation in ftrack Studio
Chris McMahon | New features, Product, Release, Studio | No Comments
Achieving Better Feedback Cycles and Faster Nuke Workflows at D-Facto Motion Studio
Kelly Messori | Case Study, Studio | No Comments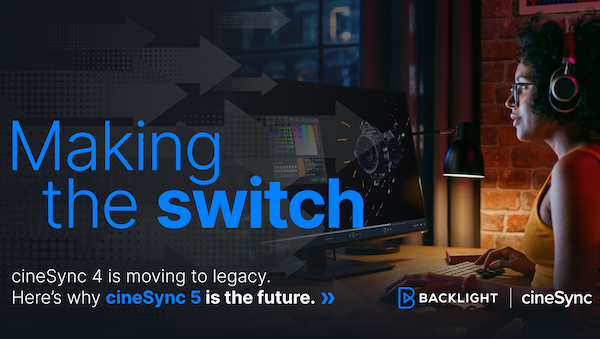
Making the switch: The transition to cineSync 5
Mahey | Announcements, cineSync, News, Product | No Comments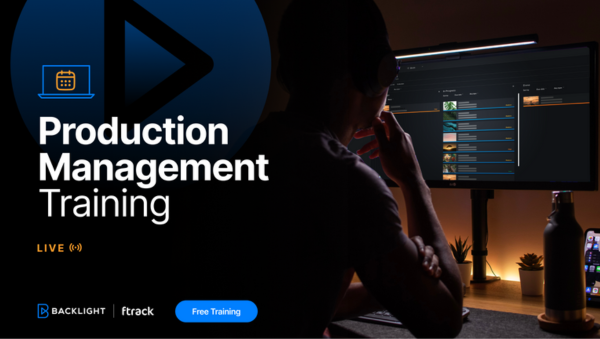
Supporting Your Studio: Free ftrack Studio Training and Office Hours from Backlight
Kelly Messori | News | No Comments
What’s new in cineSync – a deeper iconik integration, laser tool, OTIOZ support, and more
Chris McMahon | cineSync, New features, Product, Release | No Comments